CMAQ Model Evaluation Framework
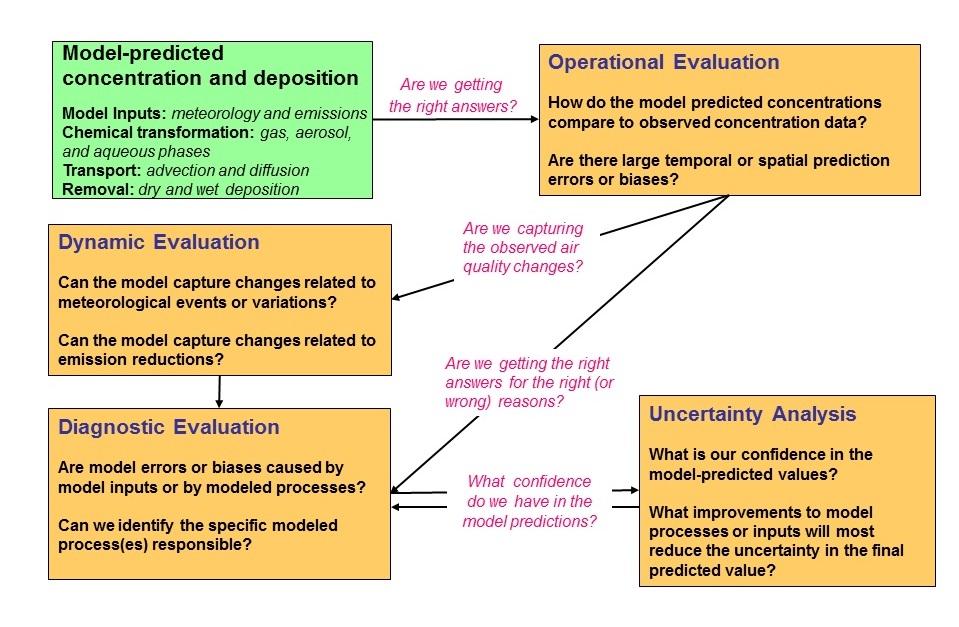
When software engineers develop a new product, whether it is a new video game, web app or business software program, the code underlying the new product is rigorously tested to identify bugs (e.g. errors in the code) and to confirm the software behaves as designed for a wide range of users. In a similar fashion, the thousands of lines of computer code that represent the “CMAQ system” have to be tested to establish the modeling system’s credibility in predicting pollutants such as ozone and particulate matter. Evaluation of the CMAQ system has been designed to assess the model’s performance for specific time periods and for specific uses of the model. For example, when CMAQ is used to support a new environmental risk assessment, the final assessment includes evaluation of the specific set of model simulations that were used.
Predictions of pollutant levels based on the CMAQ system are assessed using available observations from air quality monitors and satellites as well as laboratory and field measurements. Evaluation studies are used to not only characterize model performance at different locations and times, but also identify what model improvements are needed in the next version of the modeling system. Thus, model evaluation efforts are tied directly with model development.
The primary objectives of any CMAQ evaluation include:
- Determining if the model system is suitable for a specific application and configuration.
- Comparing the performance among different versions of the modeling system or comparing CMAQ results to other air quality models.
- Guiding model improvements.
Dennis et al. (2010) proposed a framework to describe different aspects of model evaluation under four general categories: operational, diagnostic, dynamic, and probabilistic (referred to here as uncertainty analysis).
- Operational evaluation: Are we getting the right answers?
- Operational evaluation uses statistical metrics and graphical summaries to judge how well modeled values match measurements of pollutant levels in our atmosphere. Such an analysis is always a fundamental first phase of a model evaluation study, however it is not always clear from an operational evaluation what causes the discrepancies between modeled and observed pollutant levels.
- Diagnostic evaluation: Are we getting the right answers for the right (or wrong) reasons?
- Diagnostic evaluation involves more advanced techniques to verify internal consistency within the modeling system, making sure that the connection between model inputs and outputs matches with our conceptual understanding of the underlying processes. A diagnostic evaluation is used to identify specific modeled processes responsible for model errors or biases and uncover compensating errors that can reduce the robustness of the system.
- Dynamic evaluation: Are we capturing the observed changes in air quality?
- Dynamic evaluation compares trends in model predicted pollutant levels to historical trends in observations. A key regulatory use for the model is to quantify the impact of different emission reduction strategies on pollutant concentrations. Retrospective dynamic evaluation studies are used to understand the model’s ability to predict changes due to future or hypothetical changes in emissions.
- Uncertainty analysis: What confidence do we have in model predictions?
- Uncertainty analysis quantifies the lack of knowledge and other potential sources of error in various components of the modeling system, and investigates the effect this uncertainty has on model outputs. Uncertainty analysis provides quantitative information on the confidence the model user should have in the model output. Such an analysis can also be used to establish which, among various sources of uncertainty, contributes most to the uncertainty in the final model prediction.
Since these four types of model evaluation are not necessarily mutually exclusive, research studies often incorporate aspects from more than one category of evaluation.
References
Dennis, R., Fox, T., Fuentes, M., Gilliland, A., Hanna, S., Hogrefe, C., Irwin, J., Rao, S.T., Scheffe, R., Schere, K., Steyn, D., & Venkatram, A. (2010). A framework for evaluating regional-scale numerical photo-chemical modeling systems. Environ. Fluid Mechanics, 10(4), 471–489.